- AI, Machine Learning, and Deep Learning: What They Are
- History and Development of AI
- AI Development and Its Applications
- Pros and Cons of AI
- The Future of AI
- Track AI Tokens in the Crypto.com App

AI and Its Use Cases
AI has taken the world by storm, with applications like ChatGPT and DALL-E able to produce impressive text and images. Learn more about AI and its use cases.
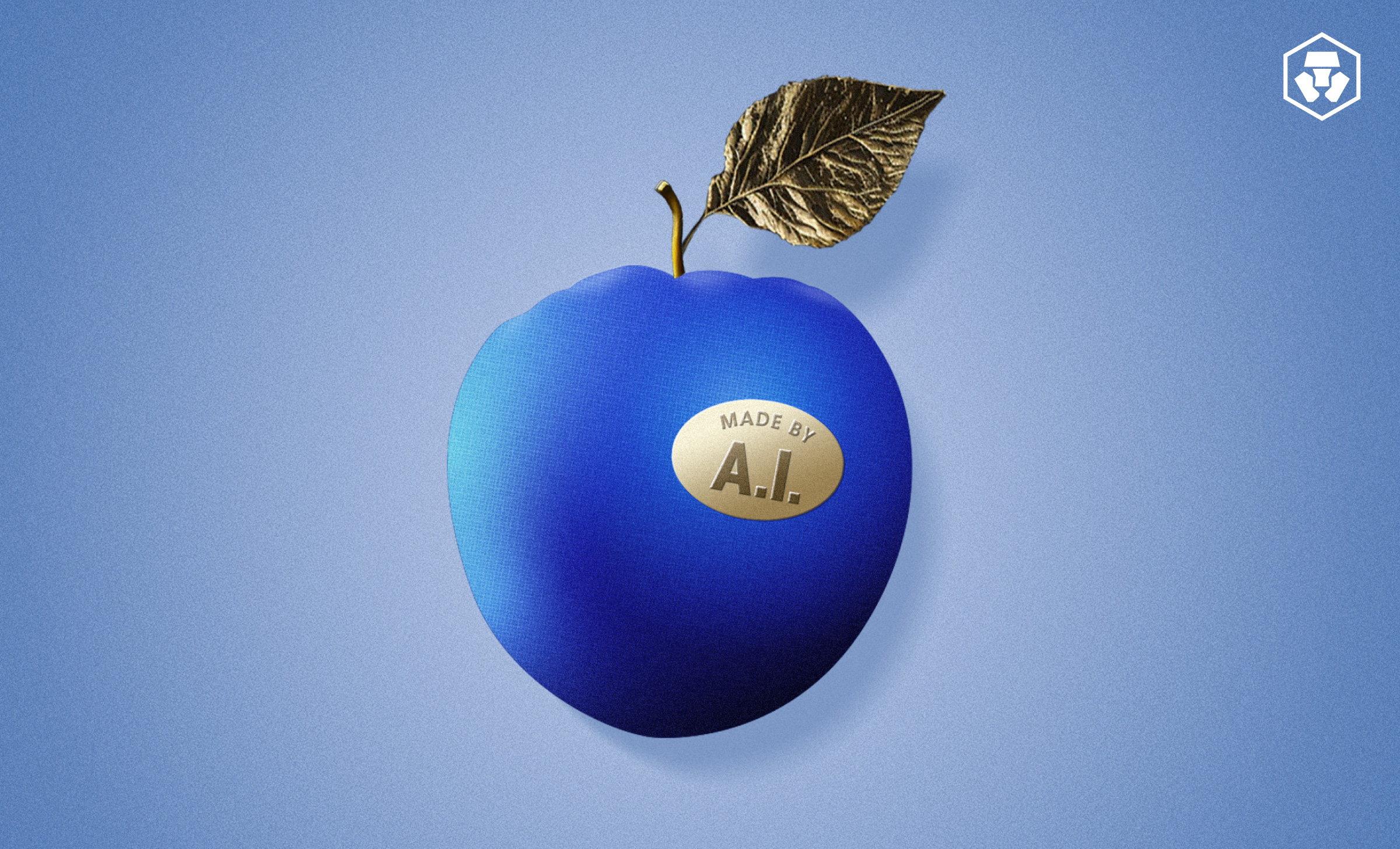
Key Takeaways:
- Artificial intelligence (AI) refers to the simulation of human intelligence in computers that are programmed to perform human-like tasks.
- Machine learning (ML) is a subset of AI that focuses on building models that can learn to use data to improve performance on a specified set of tasks.
- Deep learning is a subset of machine learning and based on artificial neural networks loosely modelled on biological neural networks in brains.
- The limited data storage and processing speed that were AI’s main drawbacks before are now no longer a major issue.
- AI has a wide variety of applications in various fields and domains. Two AI projects that have recently gained significant media attention are ChatGPT and DALL-E.
- As the amount of training data and computational power increases, it will be possible to train more powerful AI models that can lead to more effective applications.
AI, Machine Learning, and Deep Learning: What They Are
Artificial intelligence (AI) has taken the world by storm recently, together with other similar terms like machine learning (ML), deep learning, and data science. Below is a brief breakdown for those who may feel confused about these terms.
Artificial intelligence refers to the simulation of human intelligence in computers that are programmed to perform human-like tasks, such as synthesising and inferring information.
Machine learning is a subset of AI that focuses on building models that “learn,” in the sense of using data to improve performance on a specified set of tasks. Machine learning can be broadly divided into three categories:
- Supervised learning: The computer is trained on data consisting of labelled examples — known input(s) X with the ground truth output Y — and the goal is to learn a general rule or function (F) that can map inputs to outputs in new examples (i.e., Y = F(X)). An example of supervised learning is handwritten digit recognition, where the machine learns to recognise handwritten digits from 0 to 9.
- Unsupervised learning: These kinds of algorithms analyse unlabelled data to discover underlying patterns (only given X but no Y). An example of unsupervised learning is clustering, which aims to group the unlabelled data into various clusters based on their similarities or differences.
- Reinforcement learning: Reinforcement learning studies how intelligent agents should take actions in a given environment to maximise a certain reward function. Reinforcement learning can be applied to self-driving cars and robotics, as well as training machines to play board and computer games.
Deep learning is a subset of machine learning and based on artificial neural networks, which are computing systems loosely modelled on biological neural networks in brains. Artificial neurons in a network, similar to biological neurons in the brain, can receive and transmit signals to other neurons connected to it.
Data science refers to the study of data to extract meaningful insights. It is a highly interdisciplinary field that utilises statistics, mathematics, and computer science to analyse various types of data. Data science intersects with AI, machine learning, and deep learning, since artificial intelligence models typically require data for training.
The table below shows the different focuses and applications of AI, machine learning, and data science.
History and Development of AI
The current state of AI has mainly been a product of several breakthroughs in computational power over time. The limited data storage and processing speed that were AI’s main bottlenecks before are now no longer a major issue, as computing power has exponentially grown in the past decade. Hardware (like CPUs and GPUs), as well, has become cheaper and more accessible throughout the years, which has enabled the creation of new models and allowed for more funding and research for AI to come through.
Additionally, AI costs are now lower, yet training times are faster. Since 2018, training costs have decreased by 63.6%, while training times have improved by over 94%, according to Stanford’s AI Index Report. All of these developments, along with massive amounts of readily available data, have helped in training better models and yielding the better results seen today. The gradual progression of AI and some notable developments in the space are shown in the brief timeline below.
AI is not a new term, and AI algorithms like deep learning and neural networks can be dated back to the 1950s. It is only in recent years, however, that computing power has improved to make deep learning efficient, and there have also been new AI algorithms and models, such as transformers, which were introduced in 2017 by Google Brain and adopted in GPT-3, the model behind ChatGPT.
AI Development and Its Applications
AI has a wide variety of applications in various fields and domains. Two AI projects that have gained significant mainstream attention are ChatGPT and DALL-E. Both projects have experienced increased Google search interest since their launches, respectively, and have been prominently featured on social media.
ChatGPT is a chatbot that can give detailed and realistic responses to questions across wide domains of knowledge. Launched by OpenAI in November 2022, it is trained using supervised and reinforcement learning techniques. In addition to answering questions, ChatGPT can write code in various programming languages and also compose music.
DALL-E and DALL-E 2 are deep learning models that can generate images based on natural language prompts from users. The latest version of DALL-E is DALL-E 2, which was announced in April 2022. It can generate higher resolution images than DALL-E, which are more realistic and combine various styles.
Other use cases of AI include playing board games, such as Go. AlphaGo was developed by DeepMind Technologies, a subsidiary of Google, and was able to defeat Ke Jie, the No. 1-ranked Go player in the world at the time. Subsequently, AlphaGo was awarded the professional 9-dan by the Chinese Weiqi Association.
Another promising application of AI is self-driving, which enables cars to travel using reduced human input with the help of sensors to perceive their surroundings and computer systems to control and navigate the car. There are various levels of self-driving, with the current state-of-the-art technology able to reach intermediate levels like Level 3 (“eyes off”), where the driver can turn their attention away from driving.
Learn more about AI-Generated Content and Applications in Web3.
Pros and Cons of AI
Since machines can work 24 hours a day without breaks, AI is able to perform tedious and repetitive tasks more efficiently than humans. For example, AI chatbots can help to answer customers’ enquiries around the clock.
In addition, AI-powered robots and machines are able to perform tasks that are risky or dangerous for humans. For example, AI robots can explore deep oceans, defuse explosives, or work in factories with hazardous chemicals.
AI is also able to work effectively with large and high-dimensional datasets, which may be hard for humans to analyse or visualise. As computing speed increases, AI is able to perform quicker data acquisition and extraction, then transforming and interpreting the data.
One disadvantage of AI is that it sometimes lacks creativity and innovation. While AI is improving rapidly in this aspect, some of the AI-generated text and images still lack the human touch and can appear to have a rigid and robotic style.
AI can also sometimes make mistakes that a typical human would not, potentially leading to quality concerns. For example, AI researcher and Stanford professor Andrew Ng produced an example where ChatGPT erroneously explained how an abacus is faster than a GPU. Hence, in some cases, even state-of-the-art AI has yet to reach a sufficient level of accuracy.
The Future of AI
The outlook for AI is promising. According to Moore’s Law in computing, the number of transistors on a microchip doubles approximately every two years; whereas, its cost is halved over the same time frame. In short, the speed and capabilities of computers are expected to increase every two years while becoming more affordable. At the same time, the amount of data in the world is growing exponentially, projected to reach 175 zetabytes (175 trillion gigabytes) by 2025.
The above trends are highly favourable for AI because its algorithms like deep learning work more effectively with higher computing power and large amounts of data. For example, ChatGPT is classified as a Large Language Model (LLM) because it is trained on a vast quantity of text data and has 175 billion parameters. In general, as the amount of training data and computational power increases, it would be possible to train stronger AI models, which can lead to more effective applications.
There are concerns and debates that AI could lead to unemployment, since it could automate certain work tasks and achieve better performance than humans. Although AI could replace humans in tasks that require low skills like bookkeeping and data entry, the technology should be seen as a way to help humans — by automating repetitive tasks, AI can boost the productivity of employees. For instance, existing AI technologies are able to automate up to 40% of the work that salespeople perform during the sales process.
Famous physicist Stephen Hawking once said, “…computers can, in theory, emulate human intelligence — and exceed it.” In the realm of AI, this phenomenon is beginning to take shape, with computers starting to produce text and image output on par with, or even better than, that produced by the average human. It will be exciting to observe future AI developments in the years ahead.
Read the full report on AI and its use cases, including more examples and references, here.
Track AI Tokens in the Crypto.com App
The rise of AI technology has led to the emergence of new AI tokens that are quickly gaining popularity. To cater to this interest, the Crypto.com App has added a new category on Track Coins so users can easily follow the top AI tokens. Check out AI and all of the other categories here.
Due Diligence and Do Your Own Research
All examples listed in this article are for informational purposes only. You should not construe any such information or other material as legal, tax, investment, financial, or other advice. Nothing contained herein shall constitute a solicitation, recommendation, endorsement, or offer by Crypto.com to invest, buy, or sell any coins, tokens, or other crypto assets. Returns on the buying and selling of crypto assets may be subject to tax, including capital gains tax, in your jurisdiction. Any descriptions of Crypto.com products or features are merely for illustrative purposes and do not constitute an endorsement, invitation, or solicitation.
Past performance is not a guarantee or predictor of future performance. The value of crypto assets can increase or decrease, and you could lose all or a substantial amount of your purchase price. When assessing a crypto asset, it’s essential for you to do your research and due diligence to make the best possible judgement, as any purchases shall be your sole responsibility.
Share with Friends
Related Articles
From Xi Candle to Trump Pump: Bitcoin’s Legendary Trading Signals
How to Protect Against Crypto Hacks and Social Engineering
Ethereum vs Bitcoin Cash: Comparing Two Approaches for Bitcoin’s Challenges
Ready to start your crypto journey?
Get your step-by-step guide to setting upan account with Crypto.com
By clicking the Submit button you acknowledge having read the Privacy Notice of Crypto.com where we explain how we use and protect your personal data.